One day, machine learning (ML) technologies will be widely deployed across the civil service – helping civil servants to address crises such coronavirus, handling case management decisions, and providing fully automated services for citizens. But that day is still some distance away: before trusting ML to make decisions affecting the lives of citizens or employees, government bodies must build the data systems, technical expertise, regulatory frameworks and business processes to address the risks inherent in this emerging technology.
There is, however, huge value in deploying ML in less sensitive applications – realising many of the technology’s potential benefits while avoiding or addressing its weaknesses. Speaking last year, Alison Pritchard, director-general for data capability at the Office for National Statistics, argued that “supervised Machine Learning” can usefully be applied in decision-support roles, providing advice and guidance while leaving civil servants with full oversight of the source data and the responsibility for decision-making.
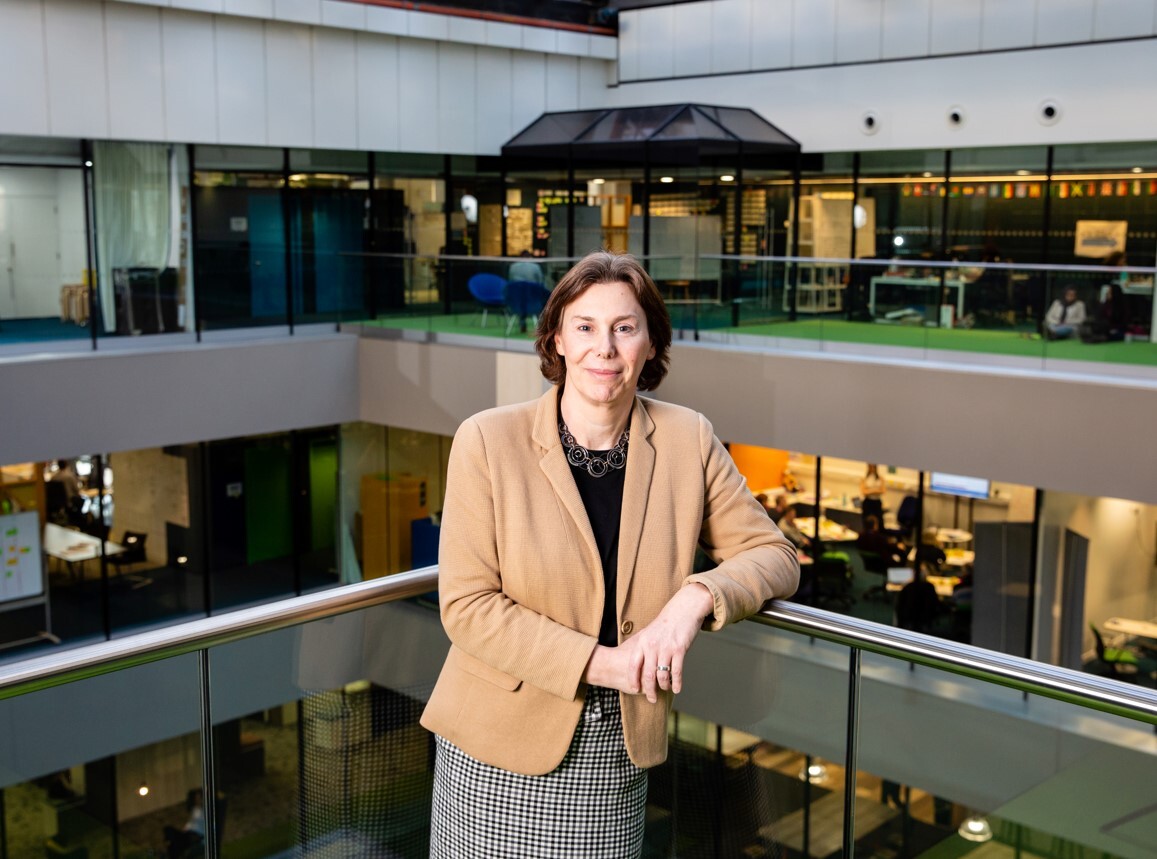
“We have a tendency to reach towards the extreme ends of these forms of technology. That impacts on our ability to seek these transformational opportunities because all of a sudden you generate all sorts of challenges,” she said. “Let’s not reach for the very end, and in the process miss opportunities to make progress in that safer space.”
In our last article we outlined the potential of ML tech and identified many of the deployment challenges to which Alison Pritchard refers – including the requirement for stronger data management and technical skills; the need to safeguard citizens’ privacy and data rights; risks to transparency, democratic accountability and regulatory structures; and the potential for ML to replicate any discriminatory outcomes evident in source data. And we highlighted the power of decision-support analytics: non-ML technologies that use fixed algorithms to comb through large datasets, guiding and informing civil servants’ work while sidestepping the challenges inherent in truly ‘learning’ systems.
Not deciding, but informing
But there is also plenty of room to deploy full ML systems within that decision-support “safer space”. Such applications require careful attention to governance arrangements, data management, staff capabilities and operating practices. Yet in our work with civil service organisations, we’ve developed ways to surmount all these obstacles – enabling government to capitalise on ML’s unique potency. For the technology’s ability to independently hone its operation, continually improving accuracy and effectiveness, puts it in a different league from earlier technologies.
Non-ML analytics systems have a powerful ability to combine and sift through vast datasets, but they can only do so according to fixed rules laid down by their programmers. So their effectiveness at launch rests on project managers’ understanding of the policy goals and the potential value to be found in the data; and to improve their effectiveness, civil servants must research potential upgrades and commission coding changes. ML systems, however, constantly refine their algorithms as data is pumped through them, learning what works from the results they obtain – and in the process spotting connections that no human could stumble across.
For example, fed with data on the hospital treatment of COVID-19 patients in health services around the world, along with information on outcomes, ML algorithms could highlight treatments likely to prove effective with particular symptoms or groups of patients. And as civil service bodies act to minimise physical contact in the delivery of public services, there are thousands more potential applications. Fed with manufacturers’ reports to environmental regulators and data on chemical spills, for example, ML algorithms could spot tell-tale signs that standards are slipping – helping regulators to focus site visits on the highest risk points.
Importantly, ML systems can also learn to spot patterns suggesting that data is flawed or misleading, giving staff a better picture of their source material’s reliability and highlighting errors that might skew decision-making. Deployed in such decision-support roles, ML allows public bodies to draw on all of public servants’ experience and expertise, while providing additional evidence-based guidance to help inform and target their work.
Overcoming obstacles
Leaving humans responsible for decision-making overcomes some of the complexities around deploying ML – including the need to retain both clear lines of accountability for the work of public bodies, and transparency over why and how decisions have been made. And with civil servants remaining in the driving seat, it’s easier to comply with the reporting and appeal processes required of ML systems by the General Data Protection Regulation (GDPR).
Nonetheless, even deploying ML in such constrained roles demands careful development, management and oversight. Subject matter experts – including frontline staff, business owners and service user groups – should play a key role in shaping their operation, working alongside data scientists and programmers. The conclusions drawn by ML systems must be rigorously audited and tested, with specialists constantly overseeing data quality and the algorithm’s evolution: blind trials can be used to compare the ML’s performance with that of human staff. And the civil servants receiving ML guidance must be trained in how to challenge and interpret the information they’re receiving, then monitored and tested: for example, flawed advice can be deliberately introduced to check that staff are watching out for ML errors.
Ideally, of course, this is the only flawed advice that staff ever receive – but ML systems are only as good as the data they process, so robust data quality management regimes are essential. These should establish careful monitoring of source data’s quality on a range of metrics; name the data stewards responsible for system oversight; ensure that officials have the data to explain decisions to the public; and identify the datasets in which errors could create significant harm – introducing quality standards that prioritise accuracy where it really matters. Just as aircraft manufacturers track and audit the supply chain providing every nut, bolt and widget used in their planes, ML managers with complete oversight of their data’s origins and handling can have far greater confidence that their solutions will fly. (For more on how to produce and deliver a data strategy, see Mark Humphries’ informative article.)
Building the platform
Finally, civil service organisations will need a range of capabilities to introduce ML safely and effectively. Some of these are ‘nice to haves’: it’s easier and cheaper to deploy ML if your data is stored in the Cloud, for example. Others, though, are essential. It is obvious that digital teams require the expertise to commission and deploy ML algorithms. But business owners, support functions and governance boards also need the skills to scrutinise and shape ML programmes, creating interdisciplinary teams to manage delivery.
So introducing ML can demand substantive changes to organisations’ underlying processes, capabilities and working methods – strengthening data handling, performance management and auditing, workforce skills, and programme management. These reforms are, of course, always good investments: every organisation needs high-quality data and the ability to manage digital projects effectively. And in time, they’ll unlock new opportunities: building the platform to introduce ML in decision-support roles creates the solid base of data, processes and skills required before organisations can consider deploying the technology in fully-automated processes.
Help from the centre
As they construct those platforms, civil service bodies should bake in adherence to the ‘FAST Track Principles’, ensuring that ML systems support Fairness, Accountability, Sustainability and Transparency. These principles ensure that, for example, decisions are unbiased and auditable, with system handlers able to explain how and why each was made. And here, advice and support from the centre is extremely valuable.
The launch in April 2018 of the Office for Artificial Intelligence was an important step in the right direction. The recent report by the Centre for Data Ethics and Innovation, which called for mandatory transparency standards, was another. The Government Digital Service has already set standards in various aspects of digital operations: a single, government-wide ML standards manual could both help civil service bodies build high-quality systems, and provide clear guidance to suppliers – fostering a market in approved products that eases procurement, supports interoperability and boosts buyer confidence.
The centre of government also has a valuable role to play in raising public understanding of ML – explaining people’s data rights, tackling ‘Skynet’ stereotypes and, crucially, demonstrating the technology’s potential to improve people’s lives. As these technologies move off the drawing board and into people’s daily lives, public acceptance will decide how far we can realise their potential to improve public services, boost economic growth and protect citizens from the health, economic and social costs of COVID-19.
In the slow roll-out of ML technologies, businesses will lead the way – taking the biggest risks, making the early investments, and finding ways around the inevitable delivery challenges. Many civil servants are rightly wary of operating at this cutting edge; until our understanding of ML’s risks and flaws is deeper, the technology should not be tasked with taking decisions that could harm the wellbeing of citizens or public servants.
Yet behind the first wave of early adopters, there is a safer space where civil service organisations can operate. And by using ML in decision-support roles, they can sidestep many of the thorniest issues around its deployment – opening up the huge potential value of the civil service’s datasets. Extracting this value demands a robust platform of good practice around data and project management, close interdisciplinary partnerships, and advanced digital skills. But by taking this road, civil service bodies can safely harness the power of ML to tackle COVID-19, achieve public policy goals, drive down costs and improve civil servants’ working lives – while laying the groundwork for the next great wave of technological innovation.
Steve Thorn is Executive Director and Richard Shreeve is Technical Director for central government at global public sector software leader Civica.
This is the second of two articles on ML; the first explained the technology’s characteristics and the challenges around deployment, highlighting the value of deploying ML in decision-support roles. Read the first article here